Kat Steele presented at the International Society of Biomechanics Technical Group on Computer Simulation in Edinburgh, Scotland on July 11, 2015. This study quantifies how traditional methods for estimating muscle activity in musculoskeletal simulation (e.g., minimizing sum of squared muscle activations) fails to accurately predict muscle activity for individuals with cerebral palsy. This research will help to guide the development of new methods to quantify patient-specific changes in neuromuscular control.
Control
Finalist for David Winter Award
Dr. Steele has been selected as one of five finalists for the David Winter Young Investigator Award at the International Society of Biomechanics. She will be presenting in the award session on Wednesday, July 15th at the conference in Glasgow. She will be presenting the results of her research on:
Altered muscle synergies during gait in cerebral palsy are not due to altered kinematics or kinetics.
Invited Speaker at Motor Control Symposium
Dr. Steele has been invited to speak at the International Society of Biomechanics’ Symposium on Motor Control in Biomechanics. Learn more about the event HERE.
MH Schwartz, A Rozumalski, KM Steele, “Dynamic motor control predicts treatment outcome for individuals with cerebral palsy,” Gait & Clinical Movement Analysis Society (Portland, OR) March 18-21, 2015.
KM Steele, MC Tresch, EJ Perreault (2015) “Consequences of biomechanically constrained tasks in the design and interpretation of synergy analyses.” Journal of Neurophysiology
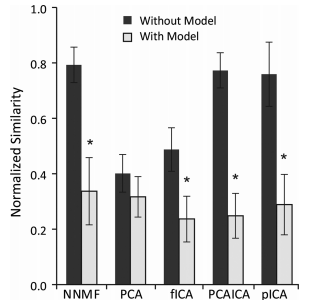
Journal article in Journal of Neurophysiology:
Consequences of biomechanically constrained tasks in the design and interpretation of synergy analyses
Matrix factorization algorithms are commonly used to analyze muscle activity and provide insight into neuromuscular control. These algorithms identify low-dimensional subspaces, commonly referred to as synergies, which can describe variation in muscle activity during a task. Synergies are often interpreted as reflecting underlying neural control; however, it is unclear how these analyses are influenced by biomechanical and task constraints, which can also lead to low-dimensional patterns of muscle activation. The aim of this study was to evaluate whether commonly used algorithms and experimental methods can accurately identify synergy-based control strategies. This was accomplished by evaluating synergies from five common matrix factorization algorithms using muscle activations calculated from 1) a biomechanically constrained task using a musculoskeletal model and 2) without task constraints using random synergy activations. Algorithm performance was assessed by calculating the similarity between estimated synergies and those imposed during the simulations; similarities ranged from 0 (random chance) to 1 (perfect similarity). Although some of the algorithms could accurately estimate specified synergies without biomechanical or task constraints (similarity >0.7), with these constraints the similarity of estimated synergies decreased significantly (0.3-0.4). The ability of these algorithms to accurately identify synergies was negatively impacted by correlation of synergy activations, which are increased when substantial biomechanical or task constraints are present. Increased variability in synergy activations, which can be captured using robust experimental paradigms that include natural variability in motor activation patterns, improved identification accuracy but did not completely overcome effects of biomechanical and task constraints. These results demonstrate that a biomechanically constrained task can reduce the accuracy of estimated synergies and highlight the importance of using experimental protocols with physiological variability to improve synergy analyses. PDF