Congratulations to Mia Hoffman for passing her General Exam!
Mia’s proposed work titled Quantifying On-Time Access to Mobility was approved by her Ph.D. committee.
Awesome job, Mia!
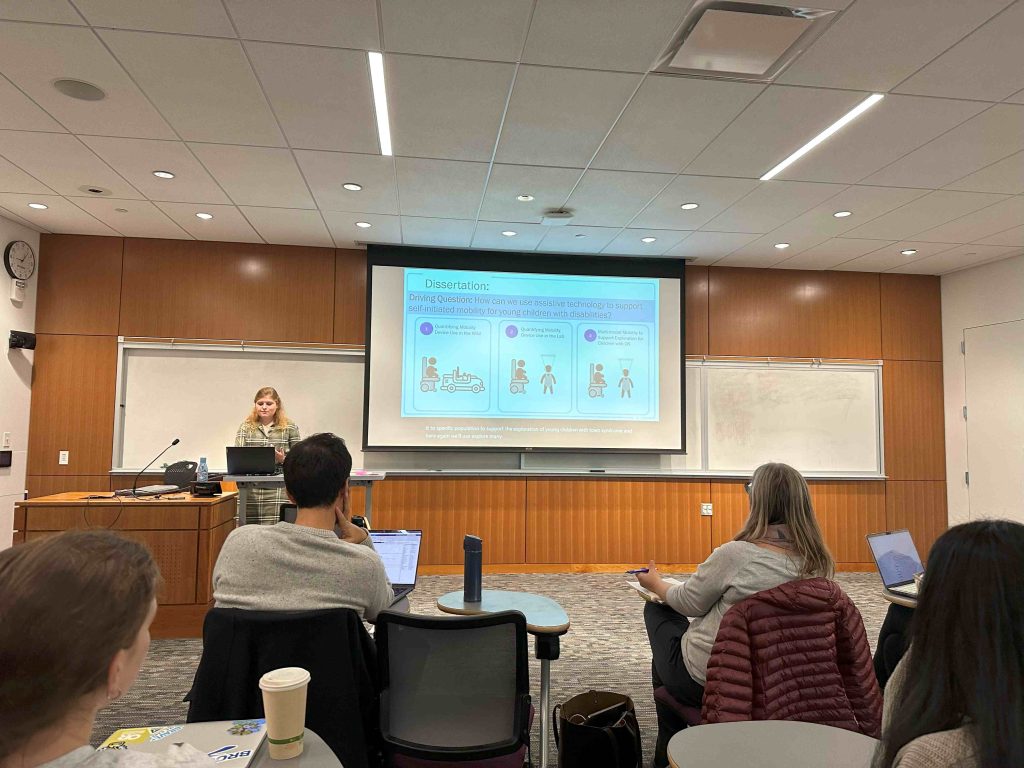
Congratulations to Mia Hoffman for passing her General Exam!
Mia’s proposed work titled Quantifying On-Time Access to Mobility was approved by her Ph.D. committee.
Awesome job, Mia!
Congratulations to Charlotte Caskey and Victoria (Tori) Landrum for presenting as finalists in the Collegiate Poster Competition at the Society of Women Engineer’s Annual Conference in LA this weekend.
Tori placed 3rd in the undergraduate student division for her poster titled “Spinal Stimulation Improves Spasticity and Motor Control in Children with Cerebral Palsy”. Charlotte placed 1st in the graduate student division for her work titled “Machine Learning for Quantifying Rehabilitation Response in Children with Cerebral Palsy.
Congratulations, Charlotte and Tori!
Lab members, Charlotte Caskey and Mia Hoffman attended the 2023 American Academy for Cerebral Palsy and Developmental Medicine (AACPDM) Annual Meeting in Chicago, IL on September 10-13, 2023.
Charlotte gave a poster presentation on “Short-Burst Interval Treadmill Training Increases Step Length and Stability for Children with Cerebral Palsy.”
Mia gave a podium presentation during the Early Detection and Diagnosis session on “Quantifying the Activity Levels of Toddlers with Down Syndrome Playing in a Partial Body Weight Support System.“
Great work in the Windy City!
This summer, the Steele Lab hosted undergraduate researcher, Amina El-Zatmah, from Santa Monica College. She finished up her 10-week summer Research Experience for Undergraduate (REU) by presenting at the 2023 Summer Undergraduate Research Symposium with the Center for Neurotechnology (CNT).
Amina gave a podium and poster presentation titled “Take A Step: The Effects of Transcutaneous Spinal Cord Stimulation and Exoskeleton Use on Step Length for Children with Cerebral Palsy“.
Amina was supported through mentorship from Charlotte Caskey, Siddhi Shrivastav, Chet Moritz, and Kat Steele.
Way to go, Amina!
Four members of our lab – Kat, Elijah, Charlotte, & Mackenzie – attended ASB 2023 on August 8-11 in Knoxville, TN.
Elijah Kuska gave a podium presentation on “The effects of weakness, contracture, and altered control on walking energetics during crouch gait.”
Charlotte Caskey gave a poster presentation on “The effect of increased sensory feedback from neuromodulation and exoskeleton use on ankle co-contraction in children with cerebral palsy.”
Kat Steele co-hosted a workshop on “Writing a Successful NIH R01 Proposal.”
ASB 2024 will be hosted August 5-8, in Madison, WI.